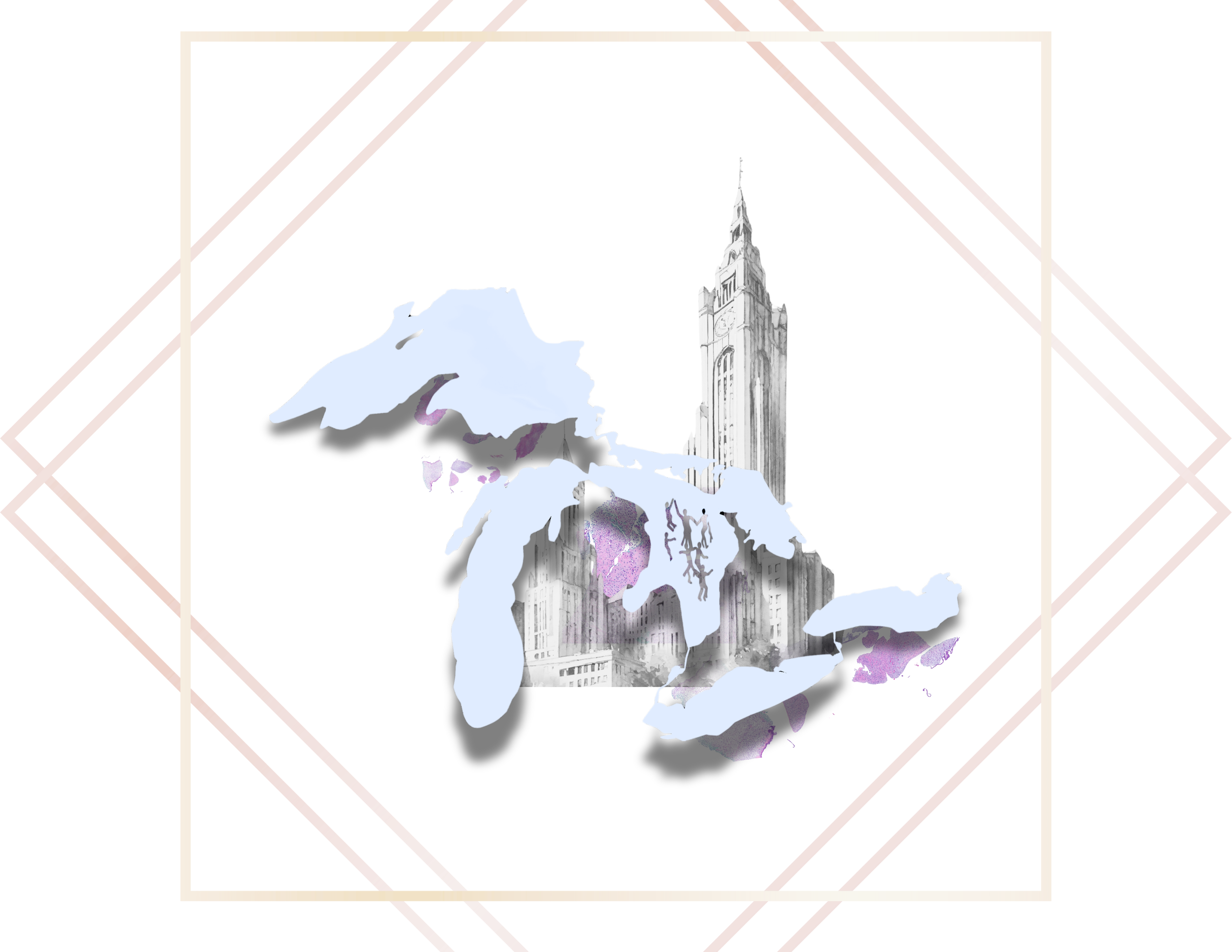
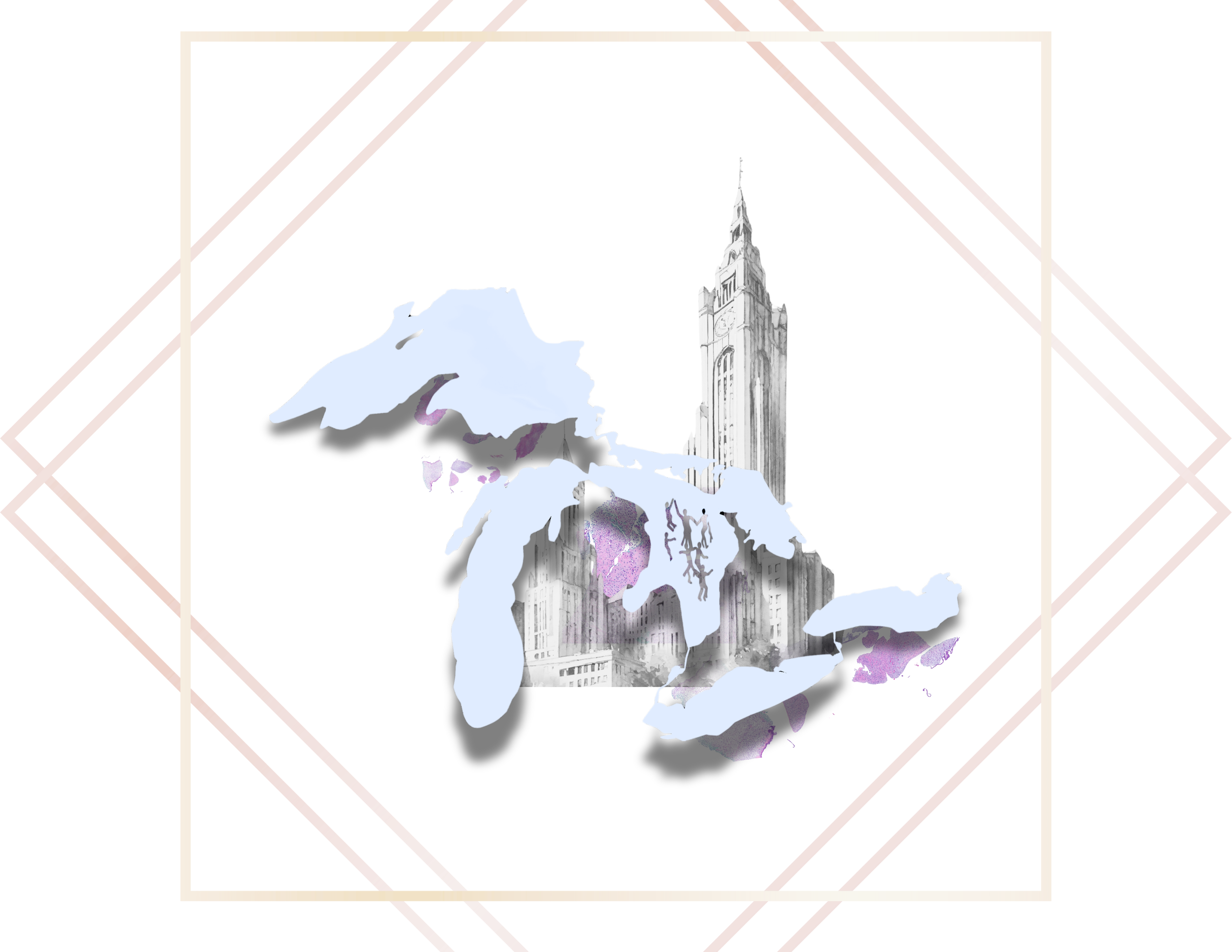
The gap between pathologists and computer scientists in digital pathology often results in a misalignment of clinical needs and computational solutions. While pathologists may hesitate to adopt computer-aided diagnostic (CAD) tools due to limited understanding of their design and purpose, computer scientists may develop technically proficient algorithms that lack clinical relevance. A standardized framework for aligning clinical goals with computational execution can bridge this divide, fostering a shared language between disciplines. This approach aims to facilitate more synergistic collaborations that advance healthcare through clinically optimized digital pathology solutions.
The integration of artificial intelligence in clinical environments transcends simple data analysis, encompassing a complex interplay of multidimensional data, technological frameworks, procedural nuances, and interdisciplinary collaboration. The Clinical AI Readiness Evaluator (CARE) framework provides a rigorous methodology for navigating and optimizing this intricate landscape, enhancing the efficacy, responsibility, and sustainability of healthcare innovations through systematic evaluation of technological readiness levels (TRL).
Biobanks operate at the intersection of personalized medicine, big data, and artificial intelligence in modern healthcare. Organizations like the Cooperative Human Tissue Network (CHTN) are uniquely positioned to advance medical research by providing high-quality, well-characterized biospecimens essential for training and validating AI models for clinical use. The management of these valuable resources presents complex challenges spanning data governance, system interoperability, and ethical considerations in human tissue collection and utilization.
The implementation of artificial intelligence (AI) in pathology practice offers transformative potential for enhancing diagnostic efficiency and accuracy. Through careful examination of unmet clinical needs, we can identify opportunities where AI technology provides meaningful solutions. The convergence of digital pathology with deep learning technologies enables both generative and non-generative AI applications, creating new possibilities for advancing diagnostic capabilities while addressing existing challenges in pathology practice.
For two centuries, pathology practice has woven quantitative observations with qualitative analysis. Pathologist language is spun from this web, encoded in a unique parlance that efficiently communicates both measurable features and their clinical implications. While current machine learning (ML) approaches excel at specific quantitative tasks, fully capturing the essence of the pathologist’s dynamic dialogue, in hopes of amplifying its impact, reveal a deeper challenge. Whether focusing on human-interpretable measurements or abstract deep-learning features, ML approaches often miss the sophisticated interplay between clinical observation and interpretation. Exploring the evolution of this practice, the path to computational solutions that enhance pathologist insights is revealed in preservation of their nuanced language.
The convergence of laboratory medicine, whole-slide imaging technology, and cloud storage creates a unique opportunity. At Mayo Clinic, state-of-the-art imaging facilities are digitizing millions of patient tissue samples, once only contained to glass slides. Digitization has enabled AI for uses such as real-time quality control. By empowering clinicians with user-friendly tools, coding experience is not required to develop algorithms used for clinical research and care. Mayo Clinic fosters inclusivity in innovation. The future of digital pathology at Mayo Clinic is multimodal. AI-driven mutation prediction algorithms and immersive VR environments designed for pathologists, by pathologists, are poised to advance diagnostic precision, efficiency, and collaboration within the medical community.